Estimating the area of buildings from satellite images
The motivation: While identifying the pixels in satellite images that depict buildings’ structures is a challenging task, it can be -at the same time- very useful for a plethora of applications. For example, tasks such as building area estimation, unused roof area availability assessment for photovoltaic installation, buildings’ density calculation or even reasoning regarding environmental matters and sustainable development.
The method: In this direction, we developed a Deep Learning (DL) model that identifies buildings based on satellite images having a 0.5meters/pixel resolution. The model, given a satellite image as input, returns as an output an image that highlights the regions that depict a building. To achieve that, we trained the DL model in an unsupervised manner on hundreds of thousands of satellite images and fine-tuned it for the specific task. The DL model can infer the area of the buildings all around the island of Cyprus, spanning an area of 9,000 sq. km.
The results: Using the output of the model, we created a GIS layer containing the footprint of the buildings in Cyprus with a precision of 94%, a recall of 99% and an Intersection over Union (IoU) metric score of 0.89. By combining the identified nadir building view provided by the model, together with information regarding the number of floors of each of the registered buildings in Cyprus provided by the Land Registry of Republic of Cyprus, we also inferred the buildings’ covered spaces area with a prediction error of less than 15% of the ground truth. These results are particularly interesting considering that we can get the buildings’ footprint and area from satellite images for any region of Cyprus with high accuracy.
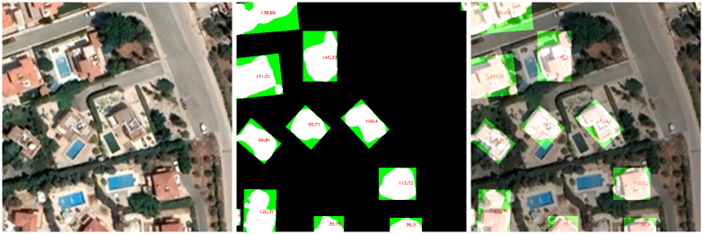
The importance: The Buildings’ Area GIS layer provides the steppingstone for high-level policy-making in environmental and landscape management aspects, for example addressing how certain residential areas are structured and the institutional frameworks governing the construction sector. It also opens a gateway to assessing a property’s compliance with local directives or state laws and the verification of the property attributes issued to state institutions and services, e.g., to land registries.
The perspective: Nevertheless, the most promising aspect of our building identification/area estimation model arises from combining its results with other facts/statistics inferred from other AI models in a data fusion framework. For example, the outcome of another model we developed that identifies swimming pools in Cyprus (PoolFinder) could be used in conjunction with the building identification results to extract useful statistics such as the correlation between the size of a building and the presence of a swimming pool in the property. We plan to explore such scenarios in the future.